Hi! I’m Kangrui Du, a first-year M.S. in Computational Science and Engineering at College of Computing, Georgia Institute of Technology. Before that, I received my Bachelor’s degree in Computer Science and Technology at University of Electronic Science and Technology of China (UESTC), where I was a member of Brain and Intelligence Lab at (UESTC) supervised by Prof. Shi Gu. I was a research intern at The Hong Kong Polytechnic University where I’m fortunate to work with Prof. Shujun Wang.
My current research interests mainly lie in building systems for fast and efficient machine learning, focusing on Spiking Neural Networks and Large Language Model acceleration. I’m also interested in programing contests and traditional algorithms, and was a member of UESTC ACM-ICPC team.
🔥 News
- 2024.8: I became a master’s student at Georgia Institute of Technology.
- 2024.6: I graduated from UESTC.
- 2024.4: I joined ByteDance as an intern, developing cloud computing systems for Douyin (TikTok China) video search.
- 2023.12: After a heated competition with the best students from various schools across the university, I’m awarded The Most Outstanding Students Award of UESTC (2023). Related link
📝 Publications
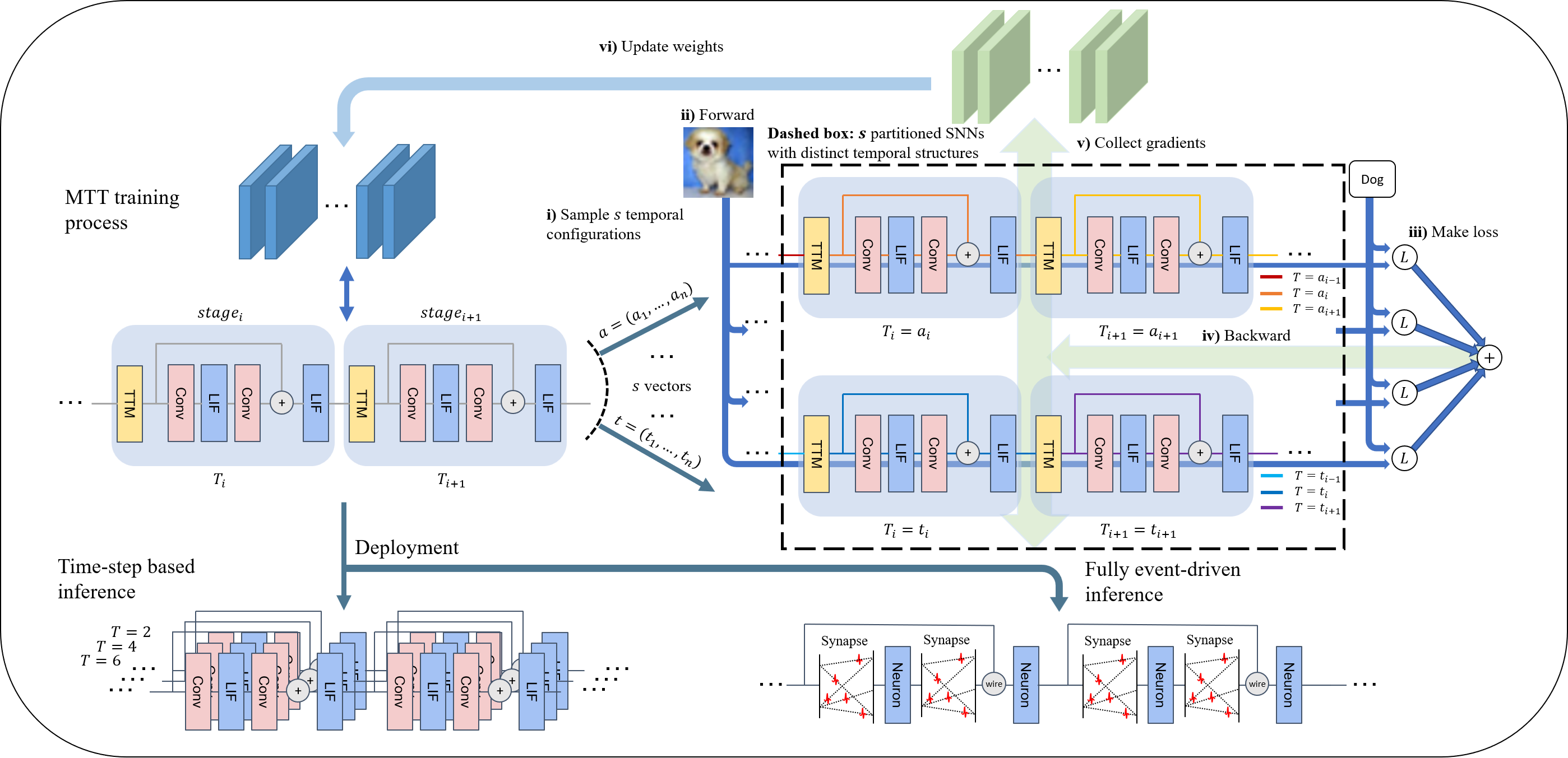
Temporal Flexibility in Spiking Neural Networks: Towards Generalization Across Time Steps and Deployment Friendliness
*Kangrui Du, *Yuhang Wu, Shikuang Deng, Shi Gu
- We identified “temporal inflexibility” caused by standard direct training, which limits SNN’s deployment on fully event-driven hardware and restricts energy-performance trade-off by dynamic inference time steps.
- We proposed MTT, a novel training approach that boosts temporal flexibility of SNN by assigning random time steps to different parts of the network during each training iteration.
- MTT’s effectiveness is proved by intensive experiments on GPU-accelerated servers, neuromorphic chips, and our high-performance event-driven simulator.
- To the best of our knowledge, our work is the first to report large model results (VGGSNN, cifar10-dvs) on fully event-driven platforms.
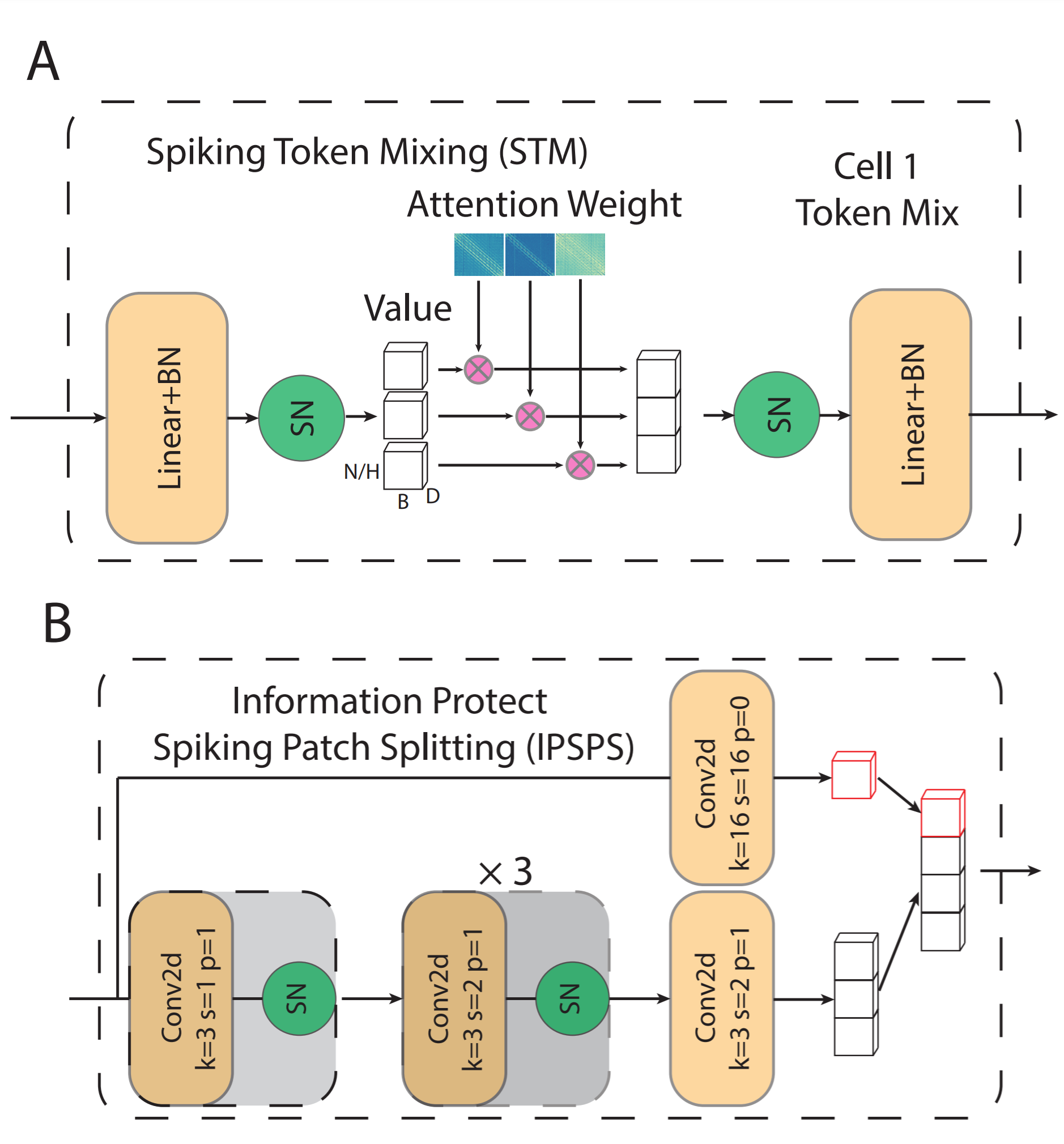
Spiking Token Mixer: An Event-Driven Friendly Former Structure for Spiking Neural Networks
*Shikuang Deng, *Yuhang Wu, Kangrui Du, Shi Gu
- To harness the energy efficiency of Spiking Neural Networks (SNNs), deploying them on neuromorphic chips is essential.
- While recent advancements have significantly boosted SNN performance, many designs remain incompatible with asynchronous, event-driven chips, limiting their integration and energy-saving potential.
- We proposed a novel state-of-the-art spike-based transformer, STMixer, to address these limitations by relying solely on operations supported by asynchronous hardware.
- Our experiments validate STMixer’s outstanding performance in both event-driven and clock-driven scenarios.
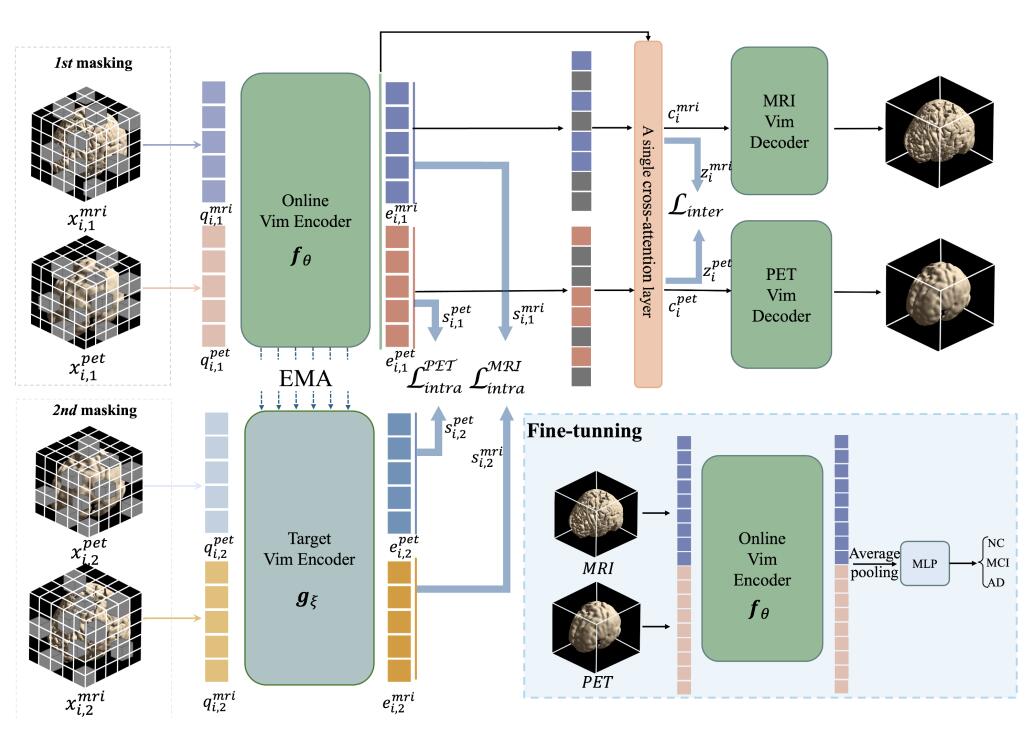
CMViM: Contrastive Masked Vim Autoencoder for 3D Multi-modal Representation Learning for AD classification
Guangqian Yang, Kangrui Du, Zhihan Yang, Ye Du, Yongping Zheng, Shujun Wang
- While many efforts were made on multimodal representation learning for medical datasets, few discussions are made to 3D medical images.
- Introduced Mamba SSM and contrastive learning in multimodal masked pre-training for 3D ViT. Our method surpassed current SOTA methods in multimodal diagnosis of Alzheimer’s Disease.
🎖 Honors and Awards
- 2023.12 The Most Outstanding Students Award of UESTC (2023) (Top 10 undergraduates in UESTC)
- 2023.10 2022-2023 China National Scholarship (top 1%)
- 2023.10 9th Place Winner in IEEEXtreme 17.0
- 2022.10 2021-2022 China National Scholarship (top 1%)
- 2022.10 4th Place Winner in IEEEXtreme 16.0
- 2021.10 2020-2021 China National Scholarship (top 1%)
- 2021.04 Gold Medal in 2020 ICPC Asia Kunming Regional Contest
- 2021.04 Silver Medal in 2020 ICPC Asia Asia-East Continent Final Contest (EC-Final)
- 2020.12 Silver Medal in 2020 ICPC Asia Shanghai Regional Contest
📖 Educations
- 2024.08 - Present, M.S. in Computational Science and Engineering, Georgia Institute of Technology (Gatech).
- 2020.09 - 2024.06, B.Eng. in Computer Science and Technology, University of Electronic Science and Technology of China (UESTC).
💻 Internships
-
2024.04 - Present, Software Engineering Intern, search architecture group at Bytedance, working on cloud computing systems for Douyin video search.
-
2021.10 - 2024.06, Research Assistant, Brain and Intelligence Lab at UESTC, working on spiking neural networks, supervised by Prof. Shi Gu.
-
2023.07 - 2024.03, Research Intern, The Hong Kong Polytechnic University, working on multimodal prognosis for Alzheimer’s Disease(AD), supervised by Prof. Shujun Wang.